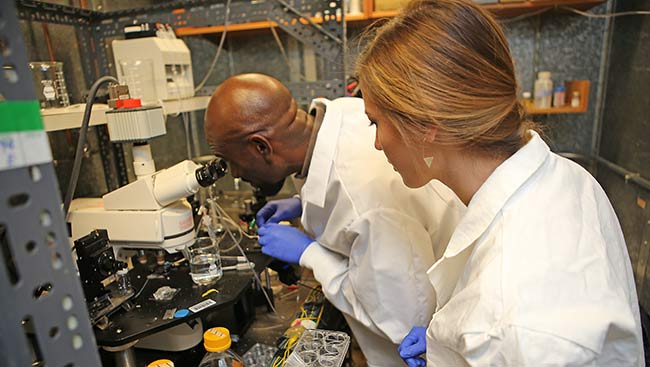
Science is about discovery. We do it because we love to learn. We always want to know if a result is true, and that involves investigating questions from many different angles to make sure a result is not a random event and that we’ve reproduced an observation over time and perhaps by different people.
But one of our natural tendencies is to look for confirmation once we are excited about an idea. We tend to ignore what doesn’t confirm our own idea because we are trying to figure out whether it seems to have general applicability.
When we investigate a problem, we want to make sure we do so with best practices. Scientific rigor is about doing the scientific method in a precise fashion. This includes designing experiments so they are well-balanced and fully executed, collecting and analyzing data in statistically valid ways, and describing what we found and how we performed the experiments when reporting results. By observing something many times, or having another person observe it, you can reproduce the observation and know it is a reliable finding.
Challenges
The challenges of scientific rigor have to do with the fact that at some level they seem invasive. As scientists, we have accomplished what we know how to do because we have an approach. But the external description of how we should do what we have been trained to do can appear top down.
Nonetheless, we have all learned how to do rigorous science. But over time, our methods can drift, and because we may be working in many areas, we may not always be doing our studies in a way that is rigorous, such as when choosing samples, designing positive or negative controls, making sure that the methods and reagents are valid and authentic, and analyzing data.
There’s also the issue of bias. It is a natural tendency to look for the information you are interested in and exclude what doesn’t work. For example, let’s say you are doing an experimental manipulation that increases neurite outgrowth. If you can identify the neurite outgrowth increase group, you will look for that. If you have another neurite non-growth group where you don’t expect that, you may not look as hard because you are not expecting it. You want to make sure you have methods in place to diminish potential for bias.
Additionally, because of changes in the professional environment and pressure to publish quickly in high-impact journals, we sometimes may be cutting corners and not employing the scientific approach we all know.
Scientific Rigor in My Lab
When performing experiments, my lab always looks to be rigorous. We go over experimental design with the students and postdocs. We ask, for instance: Do you know that technically the experiment is well designed? Do you have proper control groups? Are these reagents valid?
We also have periodic lab meetings. Because of the need for scientific rigor to be explicitly communicated in a consistent way, each student takes a topic, and once a month, we talk about them. Then everybody can say, “That is really hard for us to do, and, how can we approach that?” Understand that there are different kinds of scientific technology being used, so the discussion has to be relevant to the specific techniques.
What You Can Do
Establishing best practices for scientific rigor can be approached in several different ways.
When you design an experiment, make sure you discuss the principles of scientific rigor with your students. Ensuring the data is being collected blindly is new to many of us. You must guarantee your students and lab members cover the label of the group being analyzed. You don’t want to know the experimental control group or whether something has been treated. This allows us to reduce bias in data collection.
When planning experiments, a mentor will advise students on how to design rigorous experiments. That apprenticeship is the standard way we learn science. But buried in this process has been some of the challenges to rigor, as different individuals and relationships are not consistent over time in a laboratory. There is a need to surface scientific rigor so the methods needed for its success are consistently and broadly taught.
Graduate schools are also starting to create courses. Additionally, we expect SfN’s webinar series, Promoting Awareness and Knowledge to Enhance Scientific Rigor in Neuroscience, as part of NIH’s Training Modules to Enhance Data Reproducibility grant, of which I am a co-PI, will provide an important, consistent educational tool for students, PIs, and mentors to understand what scientific rigor is and how to accomplish it.
What is most important is that there is a broad change in the scientific community. It makes no sense that each university and PI has to learn all of this themselves. And, as of 2016, scientific rigor is part of NIH grant assessment. As a scientific community, we are all going to have to establish these methodologies and while it may seem onerous at first, it is, in fact, what we do when we are doing our best experimentation.