Data Science Approaches for Neuroscientists
- Featured in:
- Best Practices in Neuroscience Training

Neuroscientists are now collecting datasets of unprecedented scale thanks to technological advances. Yet, there are many unanswered questions that must be addressed to keep moving the field forward.
In this webinar moderated by Gardiner von Trapp, panelists Michael Miller, Richard Myers, and Pascal Wallisch discuss:
- What types of research problems can a data-science approach solve?
- How will neuroscientists analyze large-scale data sets most effectively, and with what tools?
- What training challenges do mentors and trainees face for implementing large-scale data science practices?
- What skills are valuable for neuroscientists to have to successfully understand and adopt this approach?
Watch to learn why a data-science approach is an exciting horizon in neuroscience research, and what it means for training, whether you are a PI, professor, or trainee. Then browse resources below suggested by the speakers and read Big Data: What You Should Know if you haven't already.
Michael Miller: Data Intensive Brain Science
- Kavli Neuroscience Discovery Institute at Johns Hopkins University
- Johns Hopkins Center for Imaging Science
- Neurocartography
- Map Making
- Connectomics
- Computational Functional Anatomy
- ANTS
- DARTEL
- LDDMM
- Stationary LDDMM
- Open-Source Data-Driven Tools
- Multimodal MRI Neuroinformatics
Richard Myers: Analytic Approaches for Next Generation Sequence Data
- Alzheimer’s Disease Sequencing Project (ADSP)
- Gene Expression Omnibus
- dbGaP
- Gene Expression Omnibus
- FASTX Toolkit
- SAM tools (Sequence Alignment/Map)
- Bioconductor (analysis of NGS data)
- DAVID Bioinformatics Resources 6.7
- Gene Set Enrichment Analysis (GSEA)
- Ingenuity Pathway Analysis (IPA)
Pascal Wallisch: Neural Data Science
Speakers
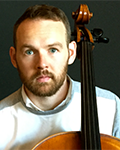
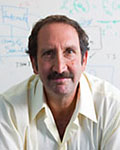
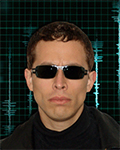
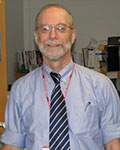